FACT Inter-dependencies: Confidentiality and Fairness
Thanks Mahnaz Qafari for the post!
Technological advances result in generating an astronomical amount of data. At the same time, it enables us to collect, store, and analyze this data. These all are done far beyond human abilities. Consequently, we as humans depend more and more on these technologies for our daily tasks. For example, we rely on Machine Learning (ML) and Artificial Intelligence (AI) for making or supporting our decisions in many areas such as credit reliability, job recommendations, healthcare, applicant selection process, etc. The more we rely on advanced techniques, the more they influence our lives, and hence, the more concerns would be raised towards their ethical issues such as fairness. When talking about the ethics in ML/AI, you hear trustworthy AI and the term FACT which is an acronym for Fairness, Accountability, Confidentiality, and Transparency where the ultimate goal is having algorithms that ensure FACT by design. However, these criteria are interconnected. In the following, we take a look at a study focusing on the inter-dependencies of these factors.
When a company wants to publish a data which includes sensitive information, they are enforced by legislation (such as EU’s General Data Protection Regulation [2]) to release privacy preserving versions of the data. In [1], the impact of using such a version of the data as input to a critical decision processes on the fairness perspective has been studied. For that, the authors has analyzed the effect of using differential private datasets as input on some resource allocation tasks. Loosely speaking, a dataset guaranties differential privacy if the probability of occurrence of an entry be similar for all possible entries regardless of its participation to the dataset. They have considered two problems:
- An allotment problem where the goal is distributing a finite set of resources among some entity. This process is shown in Figure 1 (taken from [3]). An example of such a situation is assigning a number of doctors to the healthcare system of a district.
- A decision rule such as application selection which determines whether some some benefits is granted to some entity.
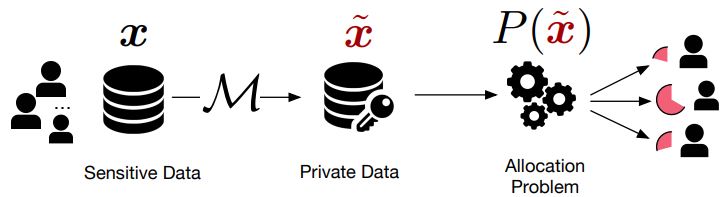
Figure 1: The private allocation process where x is a dataset, M is an epsilon-differentially private mechanism, hat(x) is the differential private version of x, and P(.) is the allotment function [3].
To characterize the effect on fairness, they have studies the distance between the expected allocation when the privacy preserved version of the dataset has been used as the input and the one where the ground truth has been used. The authors of [1] have shown that the noise added to achieve privacy disproportionately results in granting benefits or privileges to some groups over others. The authors also propose some methods to mitigate this effect:
- The output perturbation approach which suggests randomizing the outputs of the algorithms and not their inputs.
- Linearization by redundant releases which involves modifying the decision problem.
- Modified post-processing approach.
We mention an overview of a study that shows the possibility of significant societal and ethical impacts on individuals caused by using the differential private version of the data as the input to other critical algorithms. It is worth noting that such an effect has been also discussed in [4,5] as well. Here we just mentioned one of the possible impacts of the inter-dependencies of the FACT factors in ML/AI where the effect of a specific privacy-preserving technique has been studied. You are most welcome to comment your thoughts on other possible perspectives of these inter-dependencies, as well as how to prevent them.
References:
[1] https://languages.oup.com/google-dictionary-en/
[2] https://gdpr-info.eu/
[3] Tran, C., Fioretto, F., Van Hentenryck, P., Yao, Z. (2021). Decision making with differential privacy under the fairness lens. In Proceedings of the International Joint Conference on Artificial Intelligence (IJCAI).
[4] https://arxiv.org/abs/2010.04327
[5] Pujol, D., McKenna, R., Kuppam, S., Hay, M., Machanavajjhala, A., Miklau, G. (2020, January). Fair decision making using privacy-protected data. In Proceedings of the 2020 Conference on Fairness, Accountability, and Transparency (pp. 189-199).
The EU Wants to Make AI Trustworthy. Here’s What That Means for International Businesses.
Thanks Andrew Pery for the post!
On April 20, 2021 the European Commission released a much anticipated proposal for the regulation of Artificial Intelligence. The proposed regulation is intended to balance the many socio-economic benefits of AI while mitigating potential harmful impacts to fundamental human and economic rights. To achieve such a balanced approach the proposed regulation provides for a prescriptive risk-based framework to promote trustworthy AI predicated on principles of transparency, accountability, traceability, and human agency.
It is also important to point out that the EU envisions to be at the vanguard of AI innovation that delivers a fair and equitable distribution of the socio-economic potential of AI. To do so, the EU put forward a “Coordinated Plan” that includes co-funding with the private sector Testing and Experimentation Facilities for AI applications and the Digital Europe Program with an annual investment volume of € 20 billion.
We are indeed at a critical inflection point in the adoption of AI technologies that has so many beneficial applications. However, as the EU commissioned expert panel on Ethics Guidelines for Trustworthy AI so succinctly articulated:
‘Trustworthiness is a prerequisite for people and societies to develop, deploy and use AI systems. Without AI systems – and the human beings behind them – being demonstrably worthy of trust, unwanted consequences may ensue and their uptake might be hindered, preventing the realization of the potentially vast social and economic benefits that they can bring.”
Here, you will find answers to the following questions and more:
- The effort to make AI trustworthy – Who does it impact?
- Which AI systems do the proposed regulation cover?
- What are the obligations for providers of AI systems?
- What’s next?
What do you think? What are your answers to such questions? You are very welcome to write your thoughts and comments in the comments box below.
Andrew Pery is an AI Ethics Evangelist at ABBYY, a digital intelligence company. Andrew has more than 25 years of experience spearheading marketing and product management programs for leading global technology companies. His expertise is in intelligent document process automation and process intelligence with particular expertise in AI technologies, application software, data privacy, and AI ethics. He holds a Masters of Law degree with Distinction from Northwestern University Pritzker School of Law and is a Certified Data Privacy Professional.
Privacy-enhancing Technologies (PET) vs. Non-disclosure Agreements (NDA)
No one doubts that the interest in data science is growing rapidly. More and more companies are using data science and machine learning techniques to extend/accelerate their business. In the following, we provide two plots from two studies showing the growth of data science. Figure 1 is a result of a study by Towards Data Science [1] which shows the general interest in data science and other data-centric techniques by analyzing search interest trends from Google Trends years 2011-2020.
Figure 2 was taken from a study performed by the European Leadership University [2] and shows the interest in data science among software developers by analyzing the Stack Overflow survey data from 2011 to 2018.
While investments in data science and data-centric technologies grow rapidly, the responsible use of data becomes increasingly important and has the attention of consumers, citizens, and policymakers. Responsible Data Science (RDS) considers four main aspects of responsibility that need to be taken into account while analyzing data: fairness, accuracy, confidentiality, and transparency [3]. Here, we focus on the confidentiality/privacy aspect of responsible data science and invite the community to discuss the possible challenges/reasons which are prohibiting the widespread use of technical solutions, e.g., privacy-enhancing technologies (PETs), in practice.
Privacy-enhancing technologies provide data protection by eliminating or minimizing the usage of unnecessary personal data without the loss of the data utility or the functionality of an information system [4]. A non-disclosure agreement (NDA) is a legal contract between different parties that outlines confidential/private material belongs to each party and prohibits someone from sharing such confidential information.
PETs are more focused on technical solutions based on general privacy policies such as GDPR [5]. Moreover, organizations can have their own privacy/confidentiality concerns, and they may develop specific privacy preservation techniques to address such concerns. On the contrary, an NDA is only a legal contract that relies on the power of prosecutions rather than providing technical solutions. Although PETs had a lot of breakthroughs in recent years and strong technical solutions have been introduced, the companies still prefer to follow legal agreements rather than using technical solutions. The question is why technical solutions are not being widely used in practice regardless of the high demand and significant breakthroughs in academia:
- Are technical solutions still untrustworthy?
- Are they expensive to develop?
- Is there a lack of knowledge in companies to understand and develop technical solutions?
- Is the problem the lack of interpretability of technical solutions?
- Are there no solid tools to support technical solutions in practice?
Although many other possible reasons could be listed, in many cases one can still consider hybrid solutions to use technical solutions as preventative methods and use legal contracts to cover the potential weaknesses of technical solutions rather than relying on solely legal contracts which do not provide any type of technical guarantees.
What do you think? Which approach is more used in practice? And what is the main reason(s) for not using PETs in practice? You are very welcome to write your thoughts and comments in the comments box below.
References:
[1] https://towardsdatascience.com/
[2] https://elu.nl/
[3] van der Aalst W.M.P. (2017) Responsible Data Science: Using Event Data in a “People Friendly” Manner. Enterprise Information Systems. ICEIS 2016. Lecture Notes in Business Information Processing, vol 291. Springer, Cham.
[4] van Blarkom, G. W., Borking, J. J., & Olk, J. E. (2003). Handbook of privacy and privacy-enhancing technologies. Privacy Incorporated Software Agent (PISA) Consortium, The Hague, 198, 14.
[5] https://eur-lex.europa.eu/eli/reg/2016/679/oj
Contact:
We encourage experts in (responsible) data/process science to share their thoughts, experiences, and concerns regarding the responsible use of data with the community. You just need to send us your text.
Email: majid.rafiei@pads.rwth-aachen.de
Twitter: @MajidRafiei4
LinkedIn: linkedin.com/in/majid-rafiei-0838509a
PADS-RDS Welcome
Welcome to the PADS-RDS blog, where experts of (Responsible) Data Science share their thoughts, concerns, and challenges regarding the responsible use of data.