This post is by Mahnaz Qafari, Scientific Assistant in the Process And Data Science group at RWTH Aachen University. Contact her via email for further inquiries.
Processes are highly complicated entities as there are many visible and invisible parameters affecting the journey of each case in a given process. Usually there are a variety of paths taken by different cases and a variety of values assigned to their attributes even for those cases taking the same path. In such a complicated and dynamic environment, it is hard to find those friction points that deteriorate the process in terms of efficiency and other KPIs (Key Performance Indicators), and finding the reasons behind the friction points is even harder. On the other hand, re-engineering a process without this kind of information is hopeless.
The main trend for root cause analysis of an identified problem in a process is applying a machine learning technique on the data gathered from the event log of the process. But these techniques are designed for prediction, not root cause analyses. Blind usage of such results are prone to confusion between causal relationships and correlations. And acting upon them, may results in not only aggravating the current problem but also creating new ones. Having the causes of a problem diagnosed correctly, the next challenging task is anticipating and estimating the effect of changing them on the process.
There are two main frameworks to overcome these hurdles, using random experiment trial and using the theory of causality and its findings. Applying random experiment trials, i.e. randomly setting the values of those attributes that have causal effect on the problem of interest and monitoring their effect on the process, is highly expensive (and sometimes unethical if not impossible) in many situations. The other option is inferring the causal relationships between different attributes of the process using observational data and modeling these relationships by a structural causal model (also called structural equation model). Using this model, it is possible to study the effect of changes imposed by each process factor on the process indicators. Even though finding the causal structure of the process needs the aid of an expert who possess the process knowledge, process mining can benefit a lot from the theory of causality. The general approach for discovering the causal structure of a friction point in a process is depicted in Figure 1.
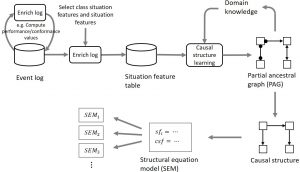
Figure 1: The general approach for structural causal equation discovery.
In our group, we aim to investigate different ways that process mining and causality inference findings can be merged, and result in more advanced and accurate process mining related techniques.