Archive for October, 2020
Columnar and Key-Value Storages in Process Mining
Object-Centric Process Mining: Dealing With Real-Life Processes
This post is by Prof. Wil M.P. van der Aalst, Chairholder of the Process And Data Science group at RWTH Aachen University. Contact him via email for further inquiries.
Techniques to discover process models from event data tend to assume precisely one case identifier per event. Unfortunately, this is often not the case. When we order from Amazon, events may refer to mixtures of orders, items, packages, customers, and products. Payments refer to orders. In one order, there may be many items (e.g., two books and three DVDs). Each of the items needs to handled separately, some may be out of stock, and others need to be moved from one warehouse to another. The same product may be ordered multiple times (in the same order or different orders). Items are combined in packages. A package may refer to multiple items from different orders and items from one order may be scattered over multiple packages. Deliveries may fail due to a variety of reasons. Hence, for one package, there may be multiple deliveries. To summarize: There are one-to-many and many-to-many relations between orders, items, packages, customers, and products. Such one-to-many and many-to-many relations between objects can be found in any process. For example, when hiring staff for multiple positions, there are applications, interviews, positions, etc. In a make-to-order company, many procurement orders may be triggered by a single sales order. Etc.
The scale of the problem becomes clear when looking at an enterprise information system like SAP. One will find many database tables related through keys implementing a one-to-many relationship between different types of business objects. There are also tables to realize many-to-many relations. Although this is common and visible for all, we still expect process models to be about individual cases. A process model may describe the life-cycle of an order or the life-cycle of an item, but typically not both. One can use swim lanes in notations like BPMN, but these are rarely used to denote one-to-many and many-to-many relationships. For sure such approaches fail to capture the above processes in a holistic manner. Object-Centric Process Mining (OCPM), one of PADS key research topics, aims to address this problem.
The usual approach to deal with the problem is to “flatten” the event data picking one of many possible case notions. There may be several candidate case notions leading to different views on the same process. As a result, one event may be related to different cases (convergence) and, for a given case, there may be multiple instances of the same activity within a case (divergence). Object-Centric Process Mining (OCPM) aims to avoid convergence and divergence problems by (1) picking a new logging format and (2) providing new process discovery techniques based on this format. This blog post summarizes part of my presentation given on 19-11-2019 in the weekly PADS Seminar Series (slides are attached).
Object-Centric Event Logs
Input for process mining is an event log. A traditional event log views a process from a particular angle provided by the case notion that is used to correlate events. Each event in such an event log refers to (1) a particular process instance (called case), (2) an activity, and (3) a timestamp. There may be additional event attributes referring to resources, people, costs, etc., but these are optional. With some effort, such data can be extracted from any information system supporting operational processes. Process mining uses these event data to answer a variety of process-related questions.
The assumption that there is just one case notion and that each event refers to precisely one case is problematic in real-life processes. Therefore, we drop the case notion and assume that an event can be related to any number of objects. In such an object-centric event log, we distinguish different order types (e.g., orders, items, packages, customers, and products). Each event has three types of attributes:
• Mandatory attributes like activity and timestamp.
• Per object type, a set of object references (zero or more per object type).
• Additional attributes (e.g., costs, etc.).
This logging format generalizes the traditional XES event logs or CSV files. A traditional event log corresponds to an object-centric event log with just one object type and one object reference per event.
Towards New Discovery Techniques
From an object-centric event log, we want to discover an object-centric process model. For example, Directly Follows Graphs (DFGs) with arcs corresponding to object types and object-centric Petri nets with places corresponding to object types. In the presentation, I described to basic approaches: One for DFGs and one for object-centric Petri nets. See the slides for more information. These baseline algorithms show that object-centric process mining is an interesting and promising research line. Alessandro Berti already implemented various discovery techniques in PM4Py-MDL leading to so-called Multiple Viewpoint Models (MVP models). Anahita Farhang also extended the ideas related to process cubes to object-centric process mining. This provides a basis for comparative process mining in a more realistic setting. An important next step is the evaluation of these ideas and implementations using more complex real-life data sets involving many object types (e.g., from SAP).
Learn More?
1. W.M.P. van der Aalst. Object-Centric Process Mining: Dealing With Divergence and Convergence in Event Data. In P.C. Ölveczky and G. Salaün, editors, Software Engineering and Formal Methods (SEFM 2019), volume 11724 of Lecture Notes in Computer Science, pages 1-23. Springer-Verlag, Berlin, 2019. https://doi.org/10.1007/978-3-030-30446-1_1
2. W.M.P. van der Aalst. A Practitioner’s Guide to Process Mining: Limitations of the Directly-Follows Graph. In International Conference on Enterprise Information Systems (Centris 2019), Procedia Computer Science, Volume 164, pages 321-328, Elsevier, 2019. https://doi.org/10.1016/j.procs.2019.12.189
3. A. Berti and W.M.P. van der Aalst. StarStar Models: Using Events at Database Level for Process Analysis. In P. Ceravolo, M.T. Gomez Lopez, and M. van Keulen, editors, International Symposium on Data-driven Process Discovery and Analysis (SIMPDA 2018), volume 2270 of CEUR Workshop Proceedings, pages 60-64. CEUR-WS.org, 2018. http://ceur-ws.org/Vol-2270/short3.pdf
4. A. Berti and W.M.P. van der Aalst. Discovering Multiple Viewpoint Models from Relational Databases. In P. Ceravolo, M.T. Gomez Lopez, and M. van Keulen, editors, Postproceedings International Symposium on Data-driven Process Discovery and Analysis, Lecture Notes in Business Information Processing. Springer-Verlag, Berlin, 2019. https://arxiv.org/abs/2001.02562
Supporting Automatic System Dynamics Model Generation for Simulation in the Context of Process Mining
This post is by Mahsa Bafrani, Scientific Assistant in the Process and Data Science team at RWTH Aachen. Contact her via email for further inquiries.
Using process mining actionable insights can be extracted from the event data stored in information systems. The analysis of event data may reveal many performance and compliance problems, and generate ideas for performance improvements. This is valuable, however, process mining techniques tend to be backward-looking and provide little support for forward-looking approaches since potential process interventions are not assessed. System dynamics complements process mining since it aims to capture the relationships between different factors at a higher abstraction level, and uses simulation to predict the effects of process improvement actions. In this paper, we propose a new approach to support the design of system dynamics models using vent data. We extract a variety of performance parameters from the current state of the process using historical execution data and provide an interactive platform for modeling the performance metrics as system dynamics models. The generated models are able to answer “what-if” questions.

Figure 1: our proposed framework for using process mining and system dynamics together.
Our proposed framework for using process mining and system dynamics together in order to design valid models to support the scenario-based prediction of business processes shown in Fig. 1. The model creation steps is an important step which we are going to focus on, i.e., the highlighted step.
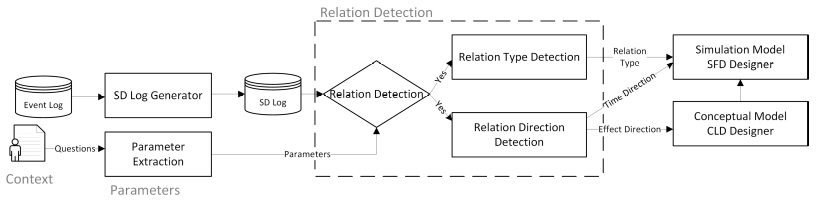
Figure 2: the main approach including the SD-log generation, relation detection, and the discovery of the type and direction of the relations.
Our approach, Fig. 2, continues with the automatic generation of causal-loop diagrams (CLD) and Stock-flow diagrams (SFD). The type of relationship is used to form the underlying equations in SFD and the effect and time directions are automatically used to design the CLD as a backbone of SFD.
In this work, we proposed a novel approach to support designing system dynamics models for simulation in the context of operational processes. Using our approach, the underlying effects and relations at the instance level can be detected and modeled in an aggregated manner. For instance, as we showed in the evaluation, the effects of the amount of workload on the speed of resources are of high importance in modeling the number of people waiting to be served per day. In the second scenario, we focused on assessing the accuracy and precision of our approach in designing a simulation model. As the evaluations show, our approach is capable of discovering hidden relations and automatically generates valid simulation models in which applying the domain knowledge is also possible. By extending the framework, we are looking to find the underlying equations between the parameters. The discovered equations help to obtain accurate simulation results in an automated fashion without user involvement. Moreover, we aim to apply the framework in case studies where we not only have the event data but can also influence the process.
Mahsa Pourbafrani, Sebastiaan J. van Zelst, Wil M. P. van der Aalst:
Supporting Automatic System Dynamics Model Generation for Simulation in the Context of Process Mining. BIS 2020: 249-263