Kategorie: ‘Allgemein’
What are Local Process Models? (and Why do They Matter?)
This post has been authored by Viki Peeva.
Local process models (LPMs) are behavioral patterns that describe process fragments and are used to analyze processes.
If we take our “definition” apart, there are three important parts regarding LPMs:
- They are a specific type of pattern.
- They do not care about the entire process but only about parts of it.
- They help us analyze and understand the process locally.
Now, let us begin!
Why are patterns important?
In data science, patterns are recurring structures or trends in the dataset. Frequent item sets and building association rules are classic examples. However, we can also consider pattern mining when we find correlations (correlation patterns), classification or clustering boundaries (separation patterns), the common ground of outliers (outlier patterns), or the famous king – man + woman = queen in word embeddings (latent patterns).
Example association rule and word embedding pattern.
Conclusion: Patterns are omnipresent in the data world, even when it does not look like that at first glance. This deduction also holds with event data. In the process mining world, we characterize patterns linked to control flow as behavioral patterns. So, next, we look at what are behavioral patterns.
What are behavioral patterns?
Consider sequential patterns, like the market basket analysis, but with time. Such sequential patterns are one example of behavioral patterns. Sequential patterns describe sequences of steps in our analyzing process [1]. If that process is buying in the supermarket, we can track what is put in the basket and in which order. That way, the supermarket might adapt product placement. However, considering hundreds or even thousands of customers visit the supermarket every day, if we try to model all their behaviors, it will probably look like a big plate of spaghetti. Hence, patterns.
Example sequential patters for buying behavior in supermarket.
The term behavioral patterns also covers patterns allowing more elaborate control-flow constructs* or defining constraints. For example, while sequences can model only sequential relationships, episodes allow concurrency modeling [2], and LPMs additionally allow choices and loops [3]. Declarative patterns allow defining constraints of what should happen first, how often, or in what order [4].
Example sequence, episode, and LPM pattern.
*Only if you are interested: These control-flow constructs are a subset of workflow patterns covered by the workflow initiative (see http://www.workflowpatterns.com/patterns/data/).
How do LPMs fit the picture?
LPMs are behavioral patterns that can model sequence, concurrency, choice, and loop. This realization covers the first important point we made at the start. In the beginning, the purpose of LPMs was the explainability of highly unstructured processes [3]. Remember the buying process in the supermarket and the thousands of customers. For these processes, traditional discovery approaches have difficulty discovering a structured process model, so they would return a spaghetti or a flower model. Hence, LPMs were supposed to have the expressiveness of traditional process models but show what happens locally and ignore the rest. However, practice has shown that LPMs are much more versatile. To prove our third point – LPMs are used to analyze processes – process miners have used them for trace encoding, event abstraction, trace classification and clustering, discovery, or in different use-case scenarios [5-8].
LPMs as replacement for flower and spaghetti process models together with various other applications like event abstraction, trace embedding, and model repair.
Nevertheless, to truly understand LPMs, we have to go back to the event log and make the connection to why LPMs describe process fragments. The simple answer is that they are patterns, but let’s dig deeper.
Let us consider the trace below. When we talk about LPMs, we look at things locally, and this locality can be as small as two events or as big as the entire process execution. Second, LPMs can ignore or skip events. Patterns do not say: “oh, excuse me, I won’t occur unless I cover everything”. No, patterns can occur hidden between events that do not matter as shown in the figure below. So when we search for them, we should be able to handle these situations. If we consider these two crucial parts together, we get to the second point of LPMs: they describe process fragments and not entire processes.
Mapping an LPM onto a trace. Locality is denoted with orange boundaries, activity mappings with blue arrows, and ignored events as question marks in red bounding box.
So far, we have covered LPMs, how they look, and their link to event logs. The next step would be discovery. Several discovery approaches exist [3,9-10], but we won’t discuss those here. We will finish with our opinion of what is next in this research area.
Where do we go from here?
Discovery. As mentioned before, multiple LPM discovery algorithms are available. All of them have strengths and weaknesses, so one way forward is to consider alternative discovery techniques or extensions of existing ones where specific weaknesses are addressed.
Pattern Explosion. On the one hand, LPM discovery, similar to any pattern discovery, suffers from the significant challenge of pattern explosion. In other words, too many patterns or LPMs are built for a human analyst to analyze. On the other hand, LPMs are versatile, meaning they can be used with different end goals in mind. Hence, the ideal solution would be to choose a unique subset of discovered LPMs that best fit the posed research question.
Conformance Checking. After discovering and choosing the optimal set of LPMs, we would like to go back to the data. Which parts of the event log do the LPMs cover? This can enable enhancing the LPMs with data perspective or specific key performance indicators (KPIs).
Much More. The world of LPMs is vast, and there’s always more to explore. If you have questions or ideas, I encourage you to share them in the comments below.
Keywords: behavioral patterns, local process models, complex models.
Icon attribution: The icons used in all figures are listed in https://github.com/VikiPeeva/SharingResources/blob/main/attribution/icons/LPMIntroPADSBlog.md
References
[1] Srikant, R., & Agrawal, R. (1996). Mining sequential patterns: Generalizations and performance improvements. In P. Apers, M. Bouzeghoub, & G. Gardarin (Eds.), Advances in Database Technology—EDBT ’96 (Vol. 1057, pp. 1–17). Springer Berlin Heidelberg. https://doi.org/10.1007/BFb0014140
[2] Leemans, M., & Van Der Aalst, W. M. P. (2015). Discovery of Frequent Episodes in Event Logs. In P. Ceravolo, B. Russo, & R. Accorsi (Eds.), Data-Driven Process Discovery and Analysis (Vol. 237, pp. 1–31). Springer International Publishing. https://doi.org/10.1007/978-3-319-27243-6_1
[3] Tax, N., Sidorova, N., Haakma, R., & Van Der Aalst, W. M. P. (2016). Mining local process models. Journal of Innovation in Digital Ecosystems, 3(2), 183–196. https://doi.org/10.1016/j.jides.2016.11.001
[4] Pesic, M., & Van Der Aalst, W. M. P. (2006). A Declarative Approach for Flexible Business Processes Management. In J. Eder & S. Dustdar (Eds.), Business Process Management Workshops (Vol. 4103, pp. 169–180). Springer Berlin Heidelberg. https://doi.org/10.1007/11837862_18
[5] Mannhardt, F., & Tax, N. (2017). Unsupervised Event Abstraction using Pattern Abstraction and Local Process Models (No. arXiv:1704.03520). arXiv. http://arxiv.org/abs/1704.03520
[6] Pijnenborg, P., Verhoeven, R., Firat, M., Laarhoven, H. V., & Genga, L. (2021). Towards Evidence-Based Analysis of Palliative Treatments for Stomach and Esophageal Cancer Patients: A Process Mining Approach. 2021 3rd International Conference on Process Mining (ICPM), 136–143. https://doi.org/10.1109/ICPM53251.2021.9576880
[7] Leemans, S. J. J., Tax, N., & Ter Hofstede, A. H. M. (2018). Indulpet Miner: Combining Discovery Algorithms. In H. Panetto, C. Debruyne, H. A. Proper, C. A. Ardagna, D. Roman, & R. Meersman (Eds.), On the Move to Meaningful Internet Systems. OTM 2018 Conferences (Vol. 11229, pp. 97–115). Springer International Publishing. https://doi.org/10.1007/978-3-030-02610-3_6
[8] Kirchner, K., & Marković, P. (2018). Unveiling Hidden Patterns in Flexible Medical Treatment Processes – A Process Mining Case Study. In F. Dargam, P. Delias, I. Linden, & B. Mareschal (Eds.), Decision Support Systems VIII: Sustainable Data-Driven and Evidence-Based Decision Support (Vol. 313, pp. 169–180). Springer International Publishing. https://doi.org/10.1007/978-3-319-90315-6_14
[9] Peeva, V., Mannel, L. L., & Van Der Aalst, W. M. P. (2022). From Place Nets to Local Process Models. In L. Bernardinello & L. Petrucci (Eds.), Application and Theory of Petri Nets and Concurrency (Vol. 13288, pp. 346–368). Springer International Publishing. https://doi.org/10.1007/978-3-031-06653-5_18
[10] Acheli, M., Grigori, D., & Weidlich, M. (2019). Efficient Discovery of Compact Maximal Behavioral Patterns from Event Logs. In P. Giorgini & B. Weber (Eds.), Advanced Information Systems Engineering (Vol. 11483, pp. 579–594). Springer International Publishing. https://doi.org/10.1007/978-3-030-21290-2_36
POSTECH Opens the “Wil van der Aalst Data & Process Science Research Center” in Pohang
Pohang University of Science and Technology (POSTECH) in South Korea has named one of its research centers after the PADS chair, prof. Wil van der Aalst. The “Wil van der Aalst Data & Process Science Research Center” reflects his significant contributions to the fields of process mining, business process management, workflow systems, and data science.
The center was opened on November 24th, 2024, on the campus of Pohang University of Science and Technology (POSTECH). A two-day symposium with speakers from industry and academia marked the starting point for the center.
POSTECH (Pohang University of Science and Technology) is a leading research university in South Korea, known for its strong focus on science, engineering, and technology. Established in 1986, it consistently ranks among the top universities globally in these fields. POSTECH is renowned for its cutting-edge research in fields like materials science, physics, chemistry, biotechnology, industrial engineering, and computer science. POSTECH has close ties with industrial partners, particularly in technology and engineering, allowing for real-world application of research. It collaborates with companies like POSCO (a major steel company) and many other global enterprises.
The “Wil van der Aalst Data & Process Science Research Center” aims to become a leading research hub, focusing on both theoretical and applied research in Data Science and Process Science. Professor Minseok Song, who initiated the establishment of the center, states that the three main goals are:
– Conducting high-impact research in Data & Process Science in collaboration with global and local partners.
– Strengthening industry-academic ties, especially in sectors such as manufacturing, service, and finance, where data and process science can drive innovation.
– Building a global research network by collaborating with distinguished international faculty and institutions.
The center is organized into three core research groups:
- Data Science Group – focusing on AI fairness, deep learning, and large-scale computational algorithms.
- Process Science Group – focusing on process mining, digital twins, and process optimization solutions.
- DPS Application Group – focused on applying research outcomes in industries such as manufacturing, finance, and insurance.
The center will play a key role in intensifying the collaboration between RWTH Aachen University and POSTECH. This will include cooperation in the field of process mining between the Process and Data Science (PADS) group at RWTH and the Department of Industrial & Management Engineering at POSTECH. The center will also facilitate student exchanges in the field of data science.
Optimization of Inventory Management in Retail Companies using Object-Centric Process Mining
This post has been authored by Dina Kretzschmann and Alessandro Berti.
Inventory management is crucial for a retails company success, as it directly impacts sales and costs. The core processes affecting inventory management are Order-to-Cash (O2C) and Purchase-to-Pay (P2P) processes. Efficiently managing these processes ensures product availability aligns with customer demand, to avoid understock (leading to lost sales) and overstock (incurring unnecessary costs) situations [1].
Current work on inventory management optimization includes (1) exact mathematical optimization models [2], (2) business management techniques [3], (3) ETL methodologies [4], and (4) traditional/object-centric process mining approaches [5]. However, gaps remain, such as the lack of standardized formalization, static assessments of key performance indicator without root cause analysis, missing links between optimization models and event data, and non-generalizable results [6].
We address these gaps by introducing a generalized object-centric data model (OCDM) for inventory management. This OCDM is enriched with relevant metrics, including Economic Order Quantity (EOQ), Reorder Point (ROP), Safety Stock (SS), Maximum Stock Level (Max), and Overstock (OS), enabling a comprehensive event-data-driven process behavior assessments and the definition of optimization measures (see Figure 1).
Figure 1 Outline of the contributions
We applied our approach to real-life O2C and P2P processes of a pet retailer utilizing the Logomate system for demand forecasting and replenishment, and SAP system for procurement and sales. The pet retailer faces issues in O2C and P2P processes leading to understock and overstock situations worth several million euros. In particular, through the standardized assessment of the interactions between different business objects we identified process behavior leading to understock and overstock situations. We quantified the frequency of these behaviors and conducted a root cause analysis, enabling the definition of optimization measures for the demand forecasting model and adjustments in the supplier contracts. The pet retailer acknowledged the added value of the results. Our approach is reproducible and generalizable with any object-centric event log following the proposed OCDM.
[1] Arnold, D., Isermann, H., Kuhn, A., Tempelmeier, H., Furmans, K.: Handbuch Logistik. Springer (2008)
[2] Tempelmeier, H.: Bestandsmanagement in supply chains. BoD–Books on Demand (2005)
[3] Rahansyah, V.Z., Kusrini, E.: How to Reduce Overstock Inventory: A Case Study. International Journal of Innovative Science and Research Techno (2023)
[4] Dong, R., Su, F., Yang, S., Xu, L., Cheng, X., Chen, W.: Design and application on metadata management for information supply chain. In: ISCIT 2021. pp. 393–396. IEEE (2016)
[5] Kretzschmann, D., Park, G., Berti, A., van der Aalst, W.M.: Overstock Problems in a Purchase-to-Pay Process: An Object-Centric Process Mining Case Study. In: CAiSE 2024 Workshops. pp. 347–359. Springer (2024)
[6] Asdecker, B., Tscherner, M., Kurringer, N., Felch, V.: A Dirty Little Secret? Conducting a Systematic Literature Review Regarding Overstocks. In: Logistics Management Conference. pp. 229–247. Springer (2023)
Sustainable Logistics Powered by Process Mining
This post has been authored by Nina Graves.
In today’s business landscape, companies are faced with the urgent need to make their processes more sustainable. Process mining techniques, known for their capability to provide valuable insights and support process improvement, are gaining increasing attention to support the transformation towards more sustainable processes [1]. To this end, we explore how process mining techniques can be enhanced to better support the transformation to more sustainable business processes. Initially, we identified the types of business processes that are most relevant for sustainability transformation: particularly production and logistics processes [2]. However, these processes are often challenging to analyse because (object-centric) process mining techniques make certain assumptions that do not always hold true:
- Every relevant process instance can be “tracked” in the event log using a unique identifier.
- The execution of an event depends on time or the “state” of the involved objects (previously executed activities, object attributes, object relationships).
- Two process executions are independent of each other.
Figure 1 – Decoupled Example Process (SP: Sub-Process)
Now imagine you are a company selling pencil cases (Figure 1):
You buy cases and pens from your suppliers (SP 1), adjust the cases and add the pens to create the final product (SP 2). Finally, you fulfil the incoming customer orders by sending the number of pencil cases you demand (SP 3). Additionally, you must ensure you always have enough pens, cases, and pencil cases to cover the incoming customer demand without keeping inventory levels too high. You would like to support your processes using PM techniques both to support your process and to analyse your scope3 emissions for the pencil cases you sell to end customers. You now face three problems: 1) You cannot detect the full end-to-end process, as there is no unique identifier for either the products you buy or the ones you sell. 2) The quantity of products you are considering varies between the sub-processes and even within the individual process executions (e.g. the demand in two customer orders is not necessarily the same). 3) You cannot consider the overall inventory management process, as it depends on the available quantities of products you cannot explicitly capture in the event log.
To bridge this gap, we are currently working on the extension of process mining techniques to support the perks of production and logistics processes. To do so, we are developing process mining techniques for the joint consideration of decoupled sub-processes [2]. Combining them with decoupling points (triangles in Figure 1), we allow for the joint consideration of (sub-)processes not combined by identifiable objects as well as another way of describing the state a process is in. This quantity state describes the count of items associated with one of the decoupling points and can be changed by executing specific events (Figure 2), e.g., “add to inventory” increases the number of products available in the incoming goods inventory.
Figure 2 – Development of the Stock Levels over Time (Quantity State)
The extension to process mining techniques we are working on allows for a more explicit consideration of quantities and their impact on the execution of events, e.g., the execution of “place replenishment order” depends on the number of pencils and cases available in the incoming goods inventory and the number of finished pencil cases. We are excited to dive deeper into this area of quantity-related process mining, as it offers many new possibilities for combining “disconnected” sub-processes and detecting quantity dependencies across multiple process executions.
References
[1] Horsthofer-Rauch, J., Guesken, S. R., Weich, J., Rauch, A., Bittner, M., Schulz, J., & Zaeh, M. F. (2024). Sustainability-integrated value stream mapping with process mining. Production & Manufacturing Research, 12(1), 2334294. https://doi.org/10.1080/21693277.2024.2334294
[2] Graves, N., Koren, I., & van der Aalst, W. M. P. (2023). ReThink Your Processes! A Review of Process Mining for Sustainability. 2023 International Conference on ICT for Sustainability (ICT4S), 164–175. https://doi.org/10.1109/ICT4S58814.2023.00025
[3] Graves, N., Koren, I., Rafiei, M., & van der Aalst, W. M. P. (2024). From Identities to Quantities: Introducing Items and Decoupling Points to Object-Centric Process Mining. In J. De Smedt & P. Soffer (Eds.), Process Mining Workshops (Vol. 503, pp. 462–474). Springer Nature Switzerland. https://doi.org/10.1007/978-3-031-56107-8_35
New Gartner Magic Quadrant for Process Mining Platforms Is Out
In 2023, Gartner published the first Magic Quadrant for Process Mining. This reflected that analyst firms started considering process mining as an important product category.
On April 29th 2024, the new Gartner Magic Quadrant for Process Mining Platforms was published. The Magic Quadrant (MQ) is a graphical tool used to evaluate technology providers, facilitating smart investment decisions through a uniform set of criteria. It categorizes providers into four types: Leaders, Visionaries, Niche Players, and Challengers. Leaders are well-executed and positioned for future success, whereas Visionaries have a clear vision of market trends but lack strong execution. Niche Players focus on specific segments without broader innovation, and Challengers perform well currently without a clear grasp of market direction.
Prof. Wil van der Aalst (c) PADS
In the 2024 MQ, five new vendors were added, and two were dropped, leading to 18 vendors being compared. Twelve additional vendors received an honorable mention. Overall, there are currently around 50 process mining vendors (see www.processmining.org). According to Gartner, “By 2026, 25% of global enterprises will have embraced process mining platforms as a first step to creating a digital twin for business operations, paving the way to autonomous business operations. Through 2026, insufficient business process management maturity will prevent 90% of organizations from reaching desired business outcomes from their end-to-end process mining initiatives.” This illustrates the relevance of the Process Mining MQ.
For the second year in a row, Celonis has been the highest ranked in terms of completeness of vision and ability to execute. Other vendors listed as leaders are Software AG, SAP Signavio, UiPath, Microsoft, Apromore, Mehrwerk, Appian, and Abbyy. The basic capabilities provided by all tools include process discovery and analysis, process comparison, analysis and validation, and discovering and validating automation opportunities. New and important capabilities include Object-Centric Process Mining (OCPM), Process-Aware Machine Learning (PAML), and Generative AI (GenAI).
For more information, download the report from https://celon.is/Gartner.
Conformance Checking Approximation Using Simulation
This post is by Mohammadreza Fani Sani, Scientific Assistant in the Process and Data Science team at RWTH Aachen. Contact her via email for further inquiries
Conformance checking techniques are used to detect deviations and to measure how accurate a process model is. Alignments were developed with the concrete goal to describe and quantify deviations in a non-ambiguous manner. Computing alignments has rapidly turned into the de facto standard conformance checking technique.
However, alignment computations may be time-consuming for real large event data. In some scenarios, the diagnostic information that is produced by alignments is not required and we simply need an objective measure of model quality to compare process models, i.e., the alignment value. Moreover, in many applications, it is required to compute alignment values several times.
As normal alignment methods take a considerable time for large real event data, analyzing many candidate process models is impractical. Therefore, by decreasing the alignment computation time, it is possible to consider more candidate process models in a limited time. Thus, by having an approximated conformance value, we can find a suitable process model faster.
By providing bounds, we guarantee that the accurate alignment value does not exceed a range of values, and, consequently we determine if it is required to do further analysis or not, which saves a considerable amount of time. Thus, in many applications, it is valuable to have a quick approximated conformance value and it is excellent worth to let users adjust the level of approximation.
In this research, we extend the previous work by proposing to use process model simulation (i.e., some of its possible executable behaviors) to create a subset of process model behaviors. The core idea of this paper is to have simulated behaviors close to the recorded behaviors in the event log. Moreover, we provide bounds for the actual conformance value.
Fig 1. A schematic view of the proposed method.
Using the proposed method, users can adjust the amount of process model behaviors considered in the approximation, which affects the computation time and the accuracy of alignment values and their bounds. As the proposed method just uses the simulated behaviors for conformance approximation, it is independent of any process model notation.
Table 1. Comparison of approximating the conformance checking using the proposed simulation method and the sampling method.
Since we use the edit distance function and do not compute any alignment, even if there is no reference process model and just some of the correct behaviors of the process (e.g., some of the valid variants) are known, the proposed method can approximate the conformance value. The method additionally returns problematic activities, based on their deviation rates.
We implemented the proposed method using both the ProM and RapidProM platforms. Moreover, we applied it to several large real event data and process models. We also compared our approach with the state-of-the-art alignment approximation method. The results show that the proposed simulation method improves the performance of the conformance checking process while providing approximations close to the actual values.
If you are interested in this research, please read the full paper at the following link: https://ieeexplore.ieee.org/document/9230162
If you need more information please contact me via fanisani@pads.rwth-aachen.de
JXES – JSON support for XES Event Logs
This post is by Madhavi Shankara Narayana, Software Engineer in the Process and Data Science team at RWTH Aachen. Contact her via email for further inquiries.
Process mining assumes the existence of an event log where each event refers to a case, an activity, and a point in time. XES is an XML based IEEE approved standard format for event logs supported by most of the process mining tools. JSON (JavaScript Object Notation), a lightweight data-interchange format has gained popularity over the last decade. Hence, we present JXES, the JSON standard for the event logs.
JXES Format
JSON is an open standard lightweight file format commonly used for data interchange. It uses human-readable text to store and transmit data objects.
For defining the JSON standard format, we have taken into account the XES meta-model represented by the basic structure (log, trace and event), Attributes, Nested Attributes, Global Attributes, Event classifiers and Extensions as shown in Figure 1.
The JXES event log structure is as shown in Figure 2.
The plugin for ProM to import and export the JSON file consists of 4 different parser implementations of import as well as export. Plugin implementations are available for Jackson, Jsoninter, GSON and simple JSON parsers.
We hope that the JXES standard defined by this paper will be helpful and serve as a guideline for generating event logs in JSON format. We also hope that the JXES standard defined in this paper will be useful for many tools like Disco, Celonis, PM4Py, etc., to enable support for JSON event logs.
For detailed information on the JXES format, please refer to https://arxiv.org/abs/2009.06363
Columnar and Key-Value Storages in Process Mining
Object-Centric Process Mining: Dealing With Real-Life Processes
This post is by Prof. Wil M.P. van der Aalst, Chairholder of the Process And Data Science group at RWTH Aachen University. Contact him via email for further inquiries.
Techniques to discover process models from event data tend to assume precisely one case identifier per event. Unfortunately, this is often not the case. When we order from Amazon, events may refer to mixtures of orders, items, packages, customers, and products. Payments refer to orders. In one order, there may be many items (e.g., two books and three DVDs). Each of the items needs to handled separately, some may be out of stock, and others need to be moved from one warehouse to another. The same product may be ordered multiple times (in the same order or different orders). Items are combined in packages. A package may refer to multiple items from different orders and items from one order may be scattered over multiple packages. Deliveries may fail due to a variety of reasons. Hence, for one package, there may be multiple deliveries. To summarize: There are one-to-many and many-to-many relations between orders, items, packages, customers, and products. Such one-to-many and many-to-many relations between objects can be found in any process. For example, when hiring staff for multiple positions, there are applications, interviews, positions, etc. In a make-to-order company, many procurement orders may be triggered by a single sales order. Etc.
The scale of the problem becomes clear when looking at an enterprise information system like SAP. One will find many database tables related through keys implementing a one-to-many relationship between different types of business objects. There are also tables to realize many-to-many relations. Although this is common and visible for all, we still expect process models to be about individual cases. A process model may describe the life-cycle of an order or the life-cycle of an item, but typically not both. One can use swim lanes in notations like BPMN, but these are rarely used to denote one-to-many and many-to-many relationships. For sure such approaches fail to capture the above processes in a holistic manner. Object-Centric Process Mining (OCPM), one of PADS key research topics, aims to address this problem.
The usual approach to deal with the problem is to “flatten” the event data picking one of many possible case notions. There may be several candidate case notions leading to different views on the same process. As a result, one event may be related to different cases (convergence) and, for a given case, there may be multiple instances of the same activity within a case (divergence). Object-Centric Process Mining (OCPM) aims to avoid convergence and divergence problems by (1) picking a new logging format and (2) providing new process discovery techniques based on this format. This blog post summarizes part of my presentation given on 19-11-2019 in the weekly PADS Seminar Series (slides are attached).
Object-Centric Event Logs
Input for process mining is an event log. A traditional event log views a process from a particular angle provided by the case notion that is used to correlate events. Each event in such an event log refers to (1) a particular process instance (called case), (2) an activity, and (3) a timestamp. There may be additional event attributes referring to resources, people, costs, etc., but these are optional. With some effort, such data can be extracted from any information system supporting operational processes. Process mining uses these event data to answer a variety of process-related questions.
The assumption that there is just one case notion and that each event refers to precisely one case is problematic in real-life processes. Therefore, we drop the case notion and assume that an event can be related to any number of objects. In such an object-centric event log, we distinguish different order types (e.g., orders, items, packages, customers, and products). Each event has three types of attributes:
• Mandatory attributes like activity and timestamp.
• Per object type, a set of object references (zero or more per object type).
• Additional attributes (e.g., costs, etc.).
This logging format generalizes the traditional XES event logs or CSV files. A traditional event log corresponds to an object-centric event log with just one object type and one object reference per event.
Towards New Discovery Techniques
From an object-centric event log, we want to discover an object-centric process model. For example, Directly Follows Graphs (DFGs) with arcs corresponding to object types and object-centric Petri nets with places corresponding to object types. In the presentation, I described to basic approaches: One for DFGs and one for object-centric Petri nets. See the slides for more information. These baseline algorithms show that object-centric process mining is an interesting and promising research line. Alessandro Berti already implemented various discovery techniques in PM4Py-MDL leading to so-called Multiple Viewpoint Models (MVP models). Anahita Farhang also extended the ideas related to process cubes to object-centric process mining. This provides a basis for comparative process mining in a more realistic setting. An important next step is the evaluation of these ideas and implementations using more complex real-life data sets involving many object types (e.g., from SAP).
Learn More?
1. W.M.P. van der Aalst. Object-Centric Process Mining: Dealing With Divergence and Convergence in Event Data. In P.C. Ölveczky and G. Salaün, editors, Software Engineering and Formal Methods (SEFM 2019), volume 11724 of Lecture Notes in Computer Science, pages 1-23. Springer-Verlag, Berlin, 2019. https://doi.org/10.1007/978-3-030-30446-1_1
2. W.M.P. van der Aalst. A Practitioner’s Guide to Process Mining: Limitations of the Directly-Follows Graph. In International Conference on Enterprise Information Systems (Centris 2019), Procedia Computer Science, Volume 164, pages 321-328, Elsevier, 2019. https://doi.org/10.1016/j.procs.2019.12.189
3. A. Berti and W.M.P. van der Aalst. StarStar Models: Using Events at Database Level for Process Analysis. In P. Ceravolo, M.T. Gomez Lopez, and M. van Keulen, editors, International Symposium on Data-driven Process Discovery and Analysis (SIMPDA 2018), volume 2270 of CEUR Workshop Proceedings, pages 60-64. CEUR-WS.org, 2018. http://ceur-ws.org/Vol-2270/short3.pdf
4. A. Berti and W.M.P. van der Aalst. Discovering Multiple Viewpoint Models from Relational Databases. In P. Ceravolo, M.T. Gomez Lopez, and M. van Keulen, editors, Postproceedings International Symposium on Data-driven Process Discovery and Analysis, Lecture Notes in Business Information Processing. Springer-Verlag, Berlin, 2019. https://arxiv.org/abs/2001.02562
Supporting Automatic System Dynamics Model Generation for Simulation in the Context of Process Mining
This post is by Mahsa Bafrani, Scientific Assistant in the Process and Data Science team at RWTH Aachen. Contact her via email for further inquiries.
Using process mining actionable insights can be extracted from the event data stored in information systems. The analysis of event data may reveal many performance and compliance problems, and generate ideas for performance improvements. This is valuable, however, process mining techniques tend to be backward-looking and provide little support for forward-looking approaches since potential process interventions are not assessed. System dynamics complements process mining since it aims to capture the relationships between different factors at a higher abstraction level, and uses simulation to predict the effects of process improvement actions. In this paper, we propose a new approach to support the design of system dynamics models using vent data. We extract a variety of performance parameters from the current state of the process using historical execution data and provide an interactive platform for modeling the performance metrics as system dynamics models. The generated models are able to answer “what-if” questions.

Figure 1: our proposed framework for using process mining and system dynamics together.
Our proposed framework for using process mining and system dynamics together in order to design valid models to support the scenario-based prediction of business processes shown in Fig. 1. The model creation steps is an important step which we are going to focus on, i.e., the highlighted step.
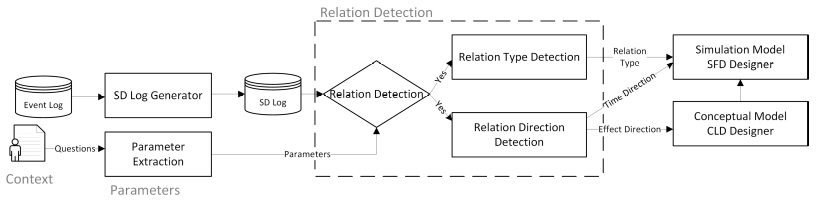
Figure 2: the main approach including the SD-log generation, relation detection, and the discovery of the type and direction of the relations.
Our approach, Fig. 2, continues with the automatic generation of causal-loop diagrams (CLD) and Stock-flow diagrams (SFD). The type of relationship is used to form the underlying equations in SFD and the effect and time directions are automatically used to design the CLD as a backbone of SFD.
In this work, we proposed a novel approach to support designing system dynamics models for simulation in the context of operational processes. Using our approach, the underlying effects and relations at the instance level can be detected and modeled in an aggregated manner. For instance, as we showed in the evaluation, the effects of the amount of workload on the speed of resources are of high importance in modeling the number of people waiting to be served per day. In the second scenario, we focused on assessing the accuracy and precision of our approach in designing a simulation model. As the evaluations show, our approach is capable of discovering hidden relations and automatically generates valid simulation models in which applying the domain knowledge is also possible. By extending the framework, we are looking to find the underlying equations between the parameters. The discovered equations help to obtain accurate simulation results in an automated fashion without user involvement. Moreover, we aim to apply the framework in case studies where we not only have the event data but can also influence the process.
Mahsa Pourbafrani, Sebastiaan J. van Zelst, Wil M. P. van der Aalst:
Supporting Automatic System Dynamics Model Generation for Simulation in the Context of Process Mining. BIS 2020: 249-263