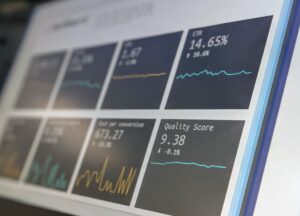
Source: Unsplash
As part of good scientific practice, research data should be stored for up to 10 years. In addition, more and more funding agencies expect information on where the collected data is stored. However, it is neither technically nor economically possible to store all data collected during a research project. Accordingly, it is necessary to carry out a data evaluation after a project has been completed. This forms the basis for deciding which data should or must be archived. Our new blog post gives a first insight into what should be considered when validating data.
Which data must be kept?
In general, the decision about what to keep depends on the priorities of the data creators. However, the decision also must take legal, regulatory or political aspects into account. These include:
- Legal or contractual reasons: Data has commercial value or is used for patent application; contractual terms or conditional states require retention.
- Policies (e.g. of institutions or funders): Disciplinary ordinances or other regulations (e.g. funding guidelines) require data retention.
- Personal data: The Data Protection Act defines personal data and sets out criteria for deciding how long it should be kept, how it must be stored and what the requirements are for disposal.
What purposes does the data serve beyond the research context?
Any of the following reasons may justify retention of the data for long-term access.
- Verification: Enabling others to understand the process that leads to published results in order to possibly reproduce or verify them.
- Further analysis: Improve the possibilities for further analysis, e.g. by using new methods.
- Further publications: Publishing a data article contributes to scientific communication and discussion about data management or reuse in your domain.
- Building an academic reputation: data that is discoverable has greater visibility, which can increase the citation rate for the published results.
- Community resource development: Publishing a data resource with value to a known group of users (e.g. reference dataset or method test bed).
- Learning & Teaching: Embedding data in a learning/teaching or public engagement resource to enhance its interactivity and motivate users to learn or participate in research.
Which data should be kept?
Considering the potential reuses previously identified, the following criteria should be considered to decide which data should be retained. As a rule, data should be retained if it meets at least two of the following criteria.
- Quality: Is the data quality good enough in terms of completeness, sample size, accuracy, validity, reliability, representativeness, or other relevant criteria?
- Integration potential: Do the data describe things that correspond to standardised terms or vocabularies in other research areas (e.g. geographical locations)?
- Interest: How likely is a demand? Could the data be of great importance, e.g. because it relates to a groundbreaking discovery, a significant new research process or international political and social concerns?
- Accessibility: Is the data in a format that does not require licensing fees or proprietary software or hardware to reuse, or is the software/hardware used widely and easily available in the field of study?
- Reproducibility: Would reproducing the data be difficult, costly or even impossible (e.g. non-reproducible observations)?
- Legal framework: Has the data been classified according to its sensitivity and is it free from any data protection, contractual restrictions, licensing or copyright provisions that limit public access and reuse?
- Unique: Is this the only and most complete copy of the data? Is the data stored somewhere where long-term storage is not guaranteed?
What costs need to be considered?
In addition, it should be weighed up whether it makes economic sense to retain the data. Consider:
- Preparation costs: Costs incurred both during the research process and in preparing for archiving.
- Storage costs: Costs incurred for storing and maintaining the data beyond the research period.
Concluding the data evaluation
The final step is to weigh the benefits against the costs, considering the results from the previous steps. Filling out a spreadsheet can help with this. Instructions can be found on the pages of the Digital Curation Centre (DCC).
Learn more
The DCC has provided a detailed Checklist for Appraising Research Data.
If you have any questions about research data management in general, feel free to write the ServiceDesk. The RDM team looks forward to hearing from you.
For more information on RDM, please visit the RWTH websites.
______
Responsible for the content of this article is Sophia Nosthoff.
Das ist ein sehr hilfreicher Artikel für den Kurationsworkflow von Forschungsdaten. Vielen Dank.
Hallo Frau Helas,
vielen Dank für Ihren netten Kommentar.
Wir freuen uns, dass wir mit dem Beitrag weiterhelfen konnten.
Liebe Grüße
das FDM-Blog-Team