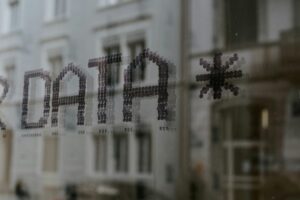
Source: Claudio Schwarz on Unsplash
In this blog post, we inform you about the origin and meaning of the so-called FAIR principles and place the term in the broader context of modern data management.
Anyone who has ever dealt with the topic of “Research Data Management” will sooner or later have come across the term “FAIR principles”. Roughly speaking, this is a rule of thumb on how research data can be made findable and exchangeable. Some of our readers will also know the meaning of the English acronym “FAIR”, which stands for “Findability, Accessibility, Interoperability, and Reusability”. But what exactly is behind it? Where does the acronym come from? What problems does it offer a solution for and who or what does it apply to?
Wilkinson’s Questions
In March 2016, the FAIR principles were published for the first time in the article “The FAIR Guiding Principles for scientific data management and stewardship” by Marc D. Wilkinson et al. Wilkinson comes from the field of medical genetics. He and other colleagues recognized that well-curated repositories for research data already existed in certain areas. Examples include Genbank, UniProt and the Worldwide Protein Data Bank (wwPDB) in the life sciences.
However, they realized that these repositories, which are highly specialized in certain data types, cannot map all data. This gave rise to numerous questions. How can data from classic experiments be mapped if the repositories only allow a single or a few formats? How is it possible to search for data for reuse in a targeted and effective way? Can the appropriate data sets be downloaded for reuse? And if so, under which license are they available?
Man and Machine: The Core Problem of Modern Data Management
Behind these questions lies a core problem of modern knowledge management, namely the barriers that prevent people from accessing data. These are primarily the huge amounts of data in a global research landscape, which present an insurmountable challenge. It can take weeks or even months to find the right data to answer research questions.
In some cases, it makes more sense to conduct the experiment yourself rather than investing time and human resources in the search, which in the worst case can end without results. So our main limitation is that we are not able to operate at the scope, scale and speed required by the volume of contemporary scientific data and its complexity. The former analog data management via archives and libraries, with their card indexes and indexes for the overview and search of data, is no longer sufficient today.
For this reason, humans are increasingly relying on computational assistance to perform data discovery and integration tasks. But there are also hurdles for machines. While it is often possible for humans to identify suitable data through contextual information, a machine relies on standardized operators to “understand” it and find and, if necessary, process it on behalf of humans. This is only successful if all data that is created globally follows common standards. And this is precisely where the FAIR principles come in. Data should be retrievable by computer and be accessible and understandable for machines and humans. This starts with the use of standardized metadata to describe the data and ends with open licenses for subsequent use. Simply “FAIR”: Findable, accessible, interoperable and reusable.
Purpose of the FAIR Principles
Do the FAIR principles solve all the challenges mentioned above? The answer to this question is a clear “yes”. The FAIR Principles serve as a guardrail along which the individual research communities should find their own paths for their subject-specific data. They explicitly do not propose any technologies, standards or implementation solutions, but help to assess the quality of research data.
They apply universally to everyone involved with research data: Repositories, publishers, universities and research institutions, infrastructure providers, researchers, managers, data stewards and so on and so forth. They can be seen as a catalyst for a fundamental cultural change in the management of data – and thus our knowledge of the world.
Practical Implementation in Germany
As digitization progressed in the European Union and consequently in Germany, it was recognized that data is valuable and that a good – FAIR – data infrastructure in research is an important factor in the modern globalized world. The FAIR principles are and were a decisive factor in the development of the specialist initiatives of the National Research Data Infrastructure (NFDI) in Germany via the German Council for Information Infrastructures (RFII) and the Joint Science Conference (GWK).
Every researcher is encouraged to make research FAIR within the scope of his or her possibilities. Our central RDM team at RWTH is happy to support you with the implementation. Please contact us by mail.
Responsible for the content of this article is Katharina Grünwald.
Leave a Reply
You must be logged in to post a comment.